ML.NET, the open-source machine learning framework for .NET developers, has just unveiled its highly anticipated version 3.0, packed with an array of new features and enhancements. This release marks a significant milestone in the evolution of ML.NET, empowering developers to seamlessly integrate custom machine learning models into their .NET applications with ease and efficiency.
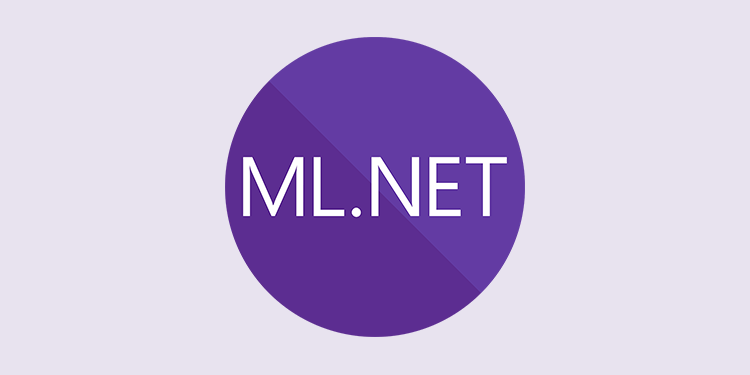
Expanding Deep Learning Capabilities
One of the most exciting aspects of the ML.NET 3.0 release is the substantial expansion of deep learning scenarios. With the integration of TorchSharp and ONNX models, developers can now leverage cutting-edge capabilities in Object Detection, Named Entity Recognition (NER), and Question Answering (QA). These advancements open up a plethora of possibilities for applications requiring advanced computer vision and natural language processing capabilities.
Object Detection
Object detection, a crucial computer vision problem, has been significantly enhanced in ML.NET 3.0. Leveraging TorchSharp-powered Object Detection APIs, developers can now perform image classification at a granular scale, accurately locating and categorizing entities within images. This feature is particularly useful in scenarios where images contain multiple objects of different types, enabling developers to build more sophisticated and intelligent applications.
Named Entity Recognition and Question Answering
Natural Language Processing (NLP) has seen remarkable advancements in the areas of Named Entity Recognition (NER) and Question Answering (QA). With ML.NET 3.0, developers can harness the power of TorchSharp RoBERTa text classification features to unlock these capabilities within their applications. The NER and QA trainers included in the release empower developers to extract valuable insights from textual data, facilitating more intelligent and context-aware applications.
Intel oneDAL Training Acceleration
ML.NET 3.0 introduces Intel oneDAL training acceleration, a groundbreaking feature that leverages highly optimized algorithmic building blocks to speed up data analysis and machine learning processes. By harnessing the power of SIMD extensions in 64-bit architectures, Intel oneDAL accelerates training tasks, enhancing the overall performance and efficiency of ML.NET applications. This integration represents a significant leap forward in training efficiency, enabling developers to train models faster and more effectively than ever before.
Automated Machine Learning (AutoML)
Automated Machine Learning (AutoML) is another key feature of ML.NET 3.0, automating the process of applying machine learning to data. With several new capabilities added to the AutoML experience, developers can now explore a wider range of machine learning scenarios. The AutoML Sweeper now supports Sentence Similarity, Question Answering, and Object Detection, expanding the scope of automated model generation. Additionally, continuous resource monitoring ensures the stability and reliability of long-running experiments, enabling developers to avoid crashes and failed trials.
DataFrame Enhancements
ML.NET 3.0 brings a plethora of enhancements to DataFrame, the versatile data manipulation tool. Community contributions, such as those from Aleksei Smirnov, have played a crucial role in improving DataFrame functionality. With support for String and VBuffer column types, increased data storage capacity, and enhanced data loading scenarios, DataFrame has become even more powerful and flexible. These enhancements streamline the data processing pipeline, empowering developers to work with large datasets more efficiently.
Integration with Tensor Primitives
ML.NET 3.0 integrates seamlessly with Tensor Primitives, a set of APIs that introduce support for tensor operations. This integration not only improves performance but also enhances the usability and functionality of ML.NET. By leveraging Tensor Primitives, developers can perform complex tensor operations with ease, unlocking new possibilities for advanced machine learning applications. Additionally, the integration serves as a valuable testing ground for the TensorPrimitives APIs, ensuring their stability and reliability in real-world scenarios.
Enhanced Integration with TorchSharp and ONNX Models
The integration with TorchSharp and ONNX models in ML.NET 3.0 opens up new avenues for developers to leverage state-of-the-art deep learning models in their applications. TorchSharp, a .NET binding to the popular PyTorch library, provides access to a vast array of pre-trained models and enables seamless interoperability between .NET and Python environments. With ONNX (Open Neural Network Exchange), developers can easily import and export models between different deep learning frameworks, facilitating collaboration and knowledge sharing within the machine learning community. By harnessing the power of TorchSharp and ONNX, developers can tap into a wealth of resources and expertise to accelerate their deep learning initiatives and build more sophisticated and intelligent applications.
Streamlined Data Processing with DataFrame
DataFrame, a core component of ML.NET, has undergone significant enhancements in version 3.0, making data processing tasks more efficient and intuitive. With support for String and VBuffer column types, developers can now work with a wider range of data formats and structures, enhancing the flexibility and versatility of DataFrame. Additionally, improvements to data loading scenarios enable seamless integration with SQL databases and other data sources, simplifying the process of importing and exporting data. These enhancements empower developers to handle large datasets with ease, enabling them to extract valuable insights and drive informed decision-making in their applications.
Community Contributions and Collaboration
The success of ML.NET 3.0 would not have been possible without the invaluable contributions and collaboration of the developer community. Community members such as Aleksei Smirnov and Andras Fuchs have played a crucial role in enhancing DataFrame functionality and implementing new features. Their dedication and expertise have enriched the ML.NET ecosystem and contributed to the overall success of the framework. Moving forward, the ML.NET team remains committed to fostering an inclusive and collaborative environment where developers from all backgrounds can contribute their ideas, insights, and expertise to drive innovation and excellence in machine learning.
Future Roadmap and Innovation
Looking ahead, the ML.NET team is already hard at work on the next iteration of the framework, with plans for .NET 9 and ML.NET 4.0 in the pipeline. As the field of machine learning continues to evolve, the team remains focused on expanding deep learning capabilities, enhancing DataFrame functionality, and integrating new APIs and technologies into the framework. With each new release, ML.NET aims to push the boundaries of what’s possible in machine learning for .NET developers, empowering them to build smarter, more efficient, and more impactful applications. Stay tuned for more updates and announcements as we continue our journey towards the future of machine learning with ML.NET.
Conclusion
In conclusion, ML.NET 3.0 represents a significant milestone in the evolution of machine learning for .NET developers. With expanded deep learning capabilities, enhanced data processing tools, and streamlined integration with cutting-edge technologies, ML.NET empowers developers to build intelligent, efficient, and scalable applications with ease. Whether you’re a seasoned machine learning practitioner or just getting started with AI development, ML.NET provides the tools, resources, and community support you need to succeed. Embrace the power of machine learning in your .NET applications and unlock a world of possibilities with ML.NET 3.0.